We now take a look at NIGnet sensitivity analysis. We add Gaussian noise to the NIGnet weight matrices and generate shapes from the resulting noisy networks.
# Basic imports
import torch
from torch import nn
import geosimilarity as gs
from NIGnets import NIGnet
from NIGnets.monotonic_nets import SmoothMinMaxNet
from assets.utils import automate_training, plot_curves
We will perform sensitivity analysis for a NIGnet that is fit to an airfoil shape. Therefore, we first fit a NIGnet to an airfoil.
from assets.shapes import airfoil
# Generate target curve points
num_pts = 1000
t = torch.linspace(0, 1, num_pts).reshape(-1, 1)
Xt = airfoil(num_pts)
nig_net = NIGnet(layer_count = 4, act_fn = nn.Tanh)
automate_training(
model = nig_net, loss_fn = gs.MSELoss(), X_train = t, Y_train = Xt,
learning_rate = 0.1, epochs = 10000, print_cost_every = 2000
)
Xc = nig_net(t)
plot_curves(Xc, Xt)
Epoch: [ 1/10000]. Loss: 0.542679
Epoch: [ 2000/10000]. Loss: 0.000147
Epoch: [ 4000/10000]. Loss: 0.000072
Epoch: [ 6000/10000]. Loss: 0.000054
Epoch: [ 8000/10000]. Loss: 0.000038
Epoch: [10000/10000]. Loss: 0.000024
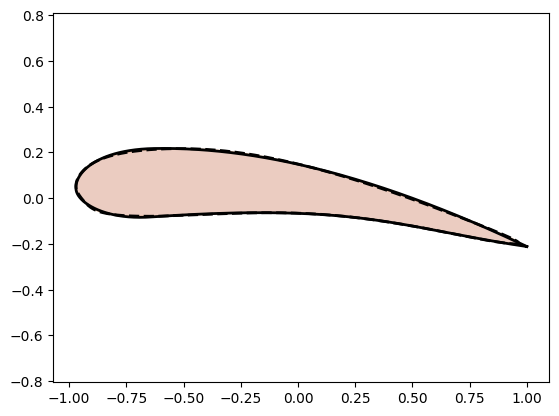
Now we use the generate_noisy_shapes()
method to produce shapes from NIGnets produced by
perturbing the weight matrices with Gaussian noise of specified standard deviation.
Every shape is produced by perturbing each weight matrix of the network as follows:
noisy_net = copy.deepcopy(self)
for param in noisy_net.parameters():
param.data += torch.randn_like(param) * noise_amount
nig_net.generate_noisy_shapes(
noise_amount = 0.02,
num_generations = 100,
num_pts = 1000
)
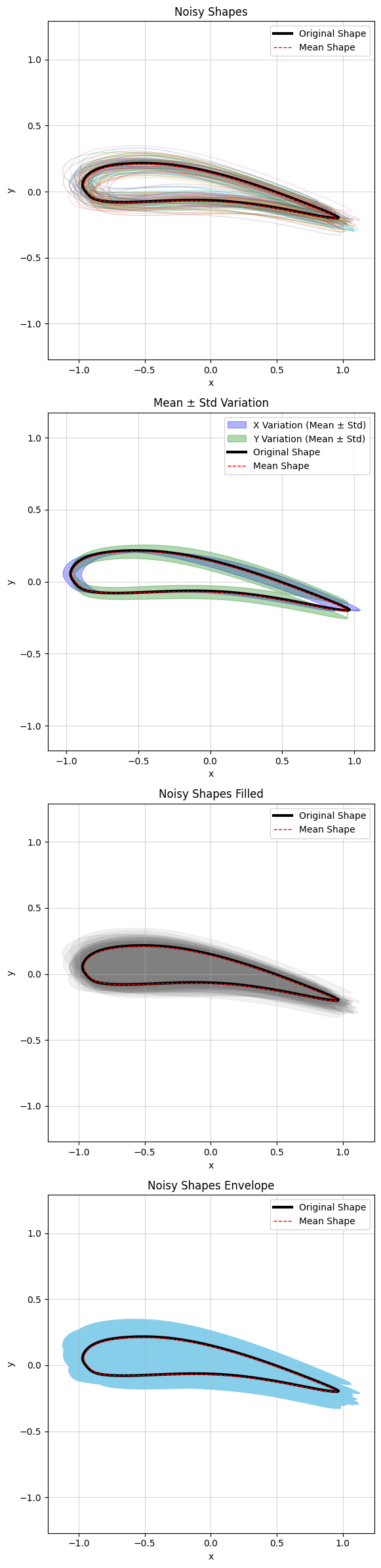